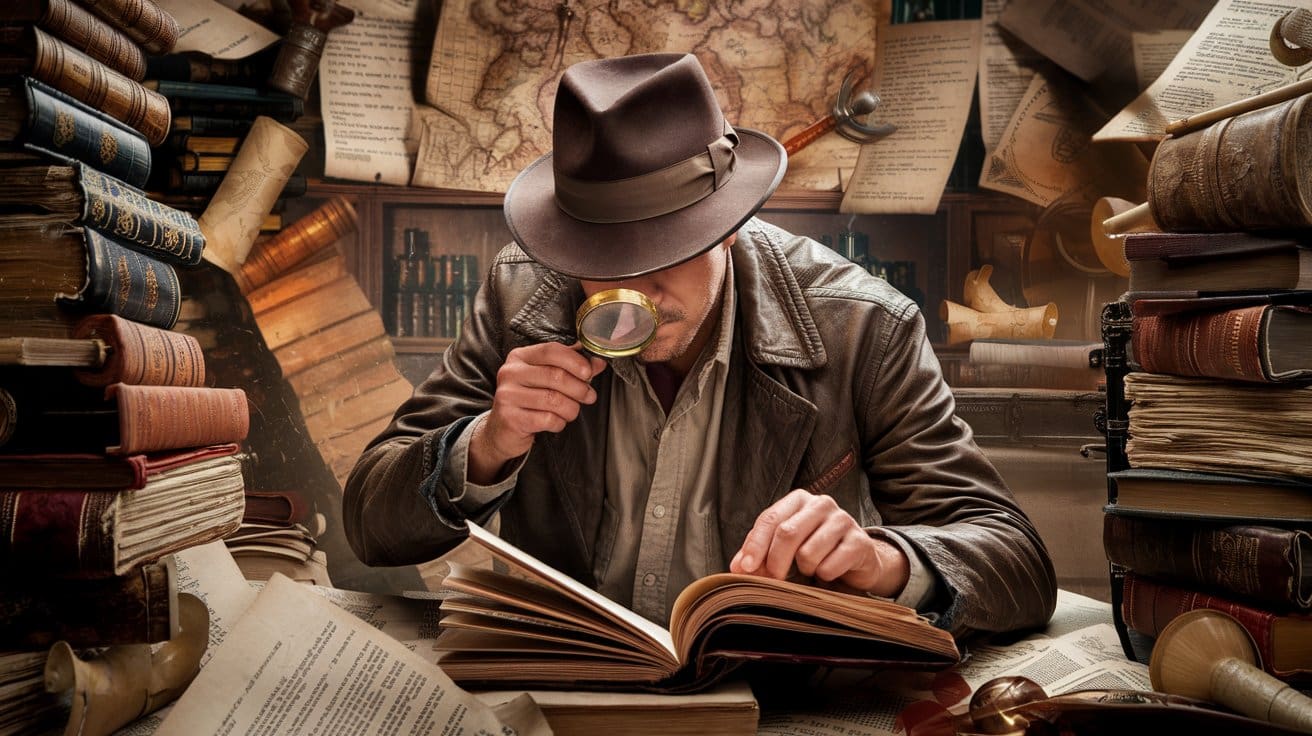
From Information to Insights: A Newbie’s Journey in Exploratory Information Evaluation
Picture by Editor | Ideogram
Each trade makes use of knowledge to make smarter choices. However uncooked knowledge might be messy and arduous to grasp. EDA permits you to discover and perceive your knowledge higher. On this article, we’ll stroll you thru the fundamentals of EDA with easy steps and examples to make it simple to observe.
What’s Exploratory Information Evaluation?
Exploratory Information Evaluation (EDA) is the method of inspecting your knowledge earlier than making a mannequin. It helps you discover patterns and spot lacking info. EDA offers you insights on tips on how to clear and put together the information. This makes positive the information is prepared for deeper evaluation and higher predictions.
Listed here are the targets of Exploratory Information Evaluation (EDA):
- Perceive Information Construction: Get a transparent image of how the information is organized and what varieties of knowledge are current.
- Establish Patterns: Search for developments or patterns that may be helpful for constructing your mannequin.
- Detect Lacking or Outlier Information: Discover any lacking or uncommon knowledge factors that might have an effect on the mannequin’s efficiency.
- Generate Preliminary Hypotheses: Provide you with assumptions concerning the knowledge that could possibly be examined later within the modeling course of.
- Summarize Key Options: Use statistics or visualizations to summarize vital features of the information.
- Information Characteristic Engineering: Use the insights from EDA to resolve tips on how to create or rework options for higher mannequin efficiency.
Steps Concerned in Exploratory Information Evaluation
Understanding the Information
Begin by understanding your dataset. Load the information and test its construction. Take a look at the varieties of variables and the general structure.
import pandas as pd import seaborn as sns import matplotlib.pyplot as plt
# Load the dataset df = pd.read_csv(‘knowledge.csv’)
# Show the primary few rows of the dataset print(“First few rows of the dataset:”) print(df.head())
# Verify the construction of the dataset print(“nStructure of the dataset:”) print(df.information()) |
Information Cleansing
Information cleansing ensures your knowledge is correct and usable. This step entails:
- Dealing with Lacking Values: Establish and handle any lacking values by filling or eradicating them.
- Eradicating Duplicates: Delete any duplicate rows to stop redundancy.
# Verify for lacking values print(df.isnull().sum())
# Drop rows with lacking values df = df.dropna()
# Take away duplicate rows df = df.drop_duplicates()
# Show the up to date dataset print(df.head()) |
Information Transformation
Reworking knowledge helps in getting ready it for evaluation. This step consists of:
- Encoding Categorical Variables: Convert categorical knowledge into numerical codecs for higher evaluation.
- Scaling Options: Regulate characteristic ranges to make sure uniformity.
from sklearn.preprocessing import LabelEncoder, StandardScaler
# One-Sizzling Encoding for categorical variables df = pd.get_dummies(df, columns=[‘Department’], drop_first=True)
# Standardizing numerical options scaler = StandardScaler() df[[‘Salary’, ‘Age’]] = scaler.fit_transform(df[[‘Salary’, ‘Age’]])
# Show the up to date dataset print(df.head()) |
Statistics Abstract
Summarizing knowledge helps you shortly perceive its primary traits and spot vital developments. Use the next strategies to get a transparent overview:
- Descriptive Statistics: Compute fundamental statistics like imply, median, commonplace deviation, and quartiles to get a way of the central tendency and unfold of numerical knowledge.
- Correlation Matrix: Consider the relationships between numerical variables to see how they’re associated to one another.
- Worth Counts: Rely the occurrences of distinctive values in categorical columns to grasp the distribution of classes.
# Descriptive statistics for numerical columns print(df.describe()) |
Univariate Evaluation
Univariate evaluation seems to be at one characteristic of the information at a time. It helps you perceive the distribution and key traits of every characteristic. This evaluation is beneficial for getting a fast overview of what every characteristic is like. Frequent strategies embody:
- Abstract Statistics: Reveals fundamental info like imply, median, and vary for numerical options.
- Histograms: Visualizes the distribution of numerical knowledge by exhibiting how usually totally different values happen.
- Boxplots: Shows the unfold of numerical knowledge and highlights outliers.
- Bar Charts: Reveals the frequency of various classes in categorical options.
For instance, you may analyze the distribution of Wage utilizing a histogram.
# Histogram for the ‘Wage’ column to test the distribution plt.hist(df[‘Salary’], bins=10, coloration=‘skyblue’) plt.title(‘Wage Distribution’) plt.xlabel(‘Wage’) plt.ylabel(‘Frequency’) plt.present() |
Bivariate Evaluation
Bivariate evaluation examines the connection between two options in your knowledge. It helps you perceive how two variables work together with one another and if they’re associated. Among the strategies embody:
- Scatter Plots: Reveals how two numerical options are associated by plotting one characteristic towards one other.
- Correlation Coefficient: Measures the energy and path of the connection between two numerical options.
- Cross-tabulation: Shows the connection between two categorical variables by exhibiting counts for every mixture of classes.
- Grouped Bar Charts: Compares the frequencies of categorical options throughout totally different teams.
For instance, you may look at the connection between Age and Wage utilizing a scatter plot.
# Scatter plot to look at the connection between ‘Age’ and ‘Wage’ plt.scatter(df[‘Age’], df[‘Salary’], coloration=‘inexperienced’) plt.title(‘Age vs Wage’) plt.xlabel(‘Age’) plt.ylabel(‘Wage’) plt.present() |
Multivariate Evaluation
Multivariate evaluation seems to be on the relationships between three or extra options on the identical time. It helps you perceive advanced interactions and patterns inside your knowledge. Strategies embody:
- Pairwise Plots: Shows scatter plots for each pair of options to point out relationships and interactions.
- Principal Element Evaluation (PCA): Reduces the variety of options by combining them into fewer, new options whereas retaining vital info.
- Correlation Matrix: Reveals the relationships between all pairs of numerical options in a grid format.
- Heatmaps: Makes use of coloration to point out the energy of relationships amongst a number of options.
For instance, you may analyze relationships between numerical variables like Age, Wage, and Bonus% utilizing a correlation matrix.
# Correlation matrix between numerical variables (Age, Wage, Bonus%) plt.determine(figsize=(8,6)) corr_matrix = df[[‘Age’, ‘Salary’, ‘Bonus %’]].corr() sns.heatmap(corr_matrix, annot=True, cmap=‘coolwarm’) plt.title(‘Correlation Matrix of Age, Wage, and Bonus%’) plt.present() |
Sensible Ideas for Efficient EDA
Listed here are some sensible tricks to observe for profitable EDA:
- Begin with a Plan: Determine what you wish to be taught out of your knowledge. This retains your evaluation organized and on observe.
- Verify Information High quality: Make certain the information is clear by fixing lacking values, duplicates, and errors. Clear knowledge results in extra correct outcomes.
- Doc Findings: Write down what you uncover. This helps you retain observe and share your insights with others.
- Search Insights: Give attention to discovering helpful info that can assist with the following steps. The purpose of EDA is to construct a robust base for additional evaluation.
Conclusion
Exploratory Information Evaluation (EDA) is a key step in understanding your knowledge. It helps you discover patterns, detect anomalies, and test knowledge high quality. By way of cleansing, remodeling, and visualizing, you achieve beneficial insights. Clear communication of those insights is vital. Use summaries, visuals, and proposals to share your findings. As you progress, you may discover superior EDA strategies.