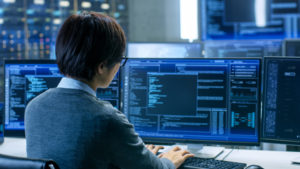
(Gorodenkoff/Shutterstock)
Information observability refers back to the skill to comprehensively monitor and perceive the conduct of knowledge inside a system. It gives transparency into real-time points of the information pipeline past information monitoring. These embrace high quality, useful resource utilization, operational metrics, system interdependencies, information lineage, and the general well being of the information infrastructure.
Within the context of knowledge integration, the power to observe and perceive the circulation of knowledge is vital to making sure the standard and reliability of knowledge because it strikes by means of varied levels of the mixing course of. With the mainstreaming of AI-powered information integration workflows, customers typically elevate reputable issues a couple of lack of transparency in workflows, information bias in reporting and analytics, or disagreements with outcomes and insights.
Strong information observability practices guarantee transparency throughout the information integration life cycle — from manufacturing to consumption — and provides enterprise customers the arrogance to make data-led enterprise choices.
Corporations with excessive information observability requirements can simply and confidently reply questions that instantly influence information integration outcomes. For instance:
- How true is the information out there to enterprise customers? Are information engineers, information scientists, and enterprise ops groups seeing and utilizing the identical information? Is our information dropping constancy in the course of the information integration course of?
- Can we observe information lineage? Do now we have a transparent document of the origins, transformations, and locations of the information because it runs by means of our pipelines? Can we replicate modifications in information integration workflows all through the information ecosystem?
- Do now we have real-time visibility into our information processes? How will modifications in a single a part of the pipeline have an effect on downstream processes? Can we detect anomalies that will influence information integrity or efficiency in real-time?
- How efficient are our root trigger evaluation processes? Do now we have quick detection of knowledge anomalies, bottlenecks and vulnerabilities that allow predictive upkeep and preventive actions?
- Can we troubleshoot successfully? When pipelines break, how shortly can we establish the point-of-failure, make a well timed intervention and repair it?
- Are our information integration workflows in compliance? Do our processes meet information governance, safety and privateness rules?
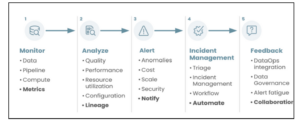
Key elements of knowledge observability (Supply: Informatica)
Whereas bottlenecks and breakages can happen even with the very best of knowledge pipelines, observability places in place checkpoints to convey belief and credibility to the information. Finally, the extra the enterprise trusts and makes use of the information, the upper the ROI of knowledge integration investments.
AI-Powered Information Observability
In more and more advanced, hybrid information integration environments, the necessity for information observability practices is extra pressing than ever. Nevertheless handbook processes are woefully insufficient to handle these calls for.
AI-driven instruments enhance information observability and supply real-time visibility into information pipelines by automating the monitoring, evaluation, and detection of points throughout workflows, regardless of how large-scale and sophisticated the operations.
Some areas the place AI-driven instruments make a big influence embrace:
Anomaly Detection
In advanced information integration environments, even figuring out the point-of-failure in a pipeline is usually a problem. AI algorithms can study the traditional patterns and behaviors of knowledge flows and flag any anomalies or deviations from these patterns. Trendy AI-powered information observability instruments assist cut back the mean-time-to-detect (MTTD) and meantime-to-resolve (MTTR) information high quality and pipeline issues.
Predictive Analytics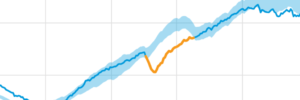
Machine studying fashions assist predict future tendencies or points primarily based on historic information patterns. This visibility helps predict potential bottlenecks, latency points, or errors in information integration processes, permitting for proactive optimization and ongoing course of enchancment.
Automated Root-Trigger Evaluation
AI can analyze in depth information and system logs to establish the foundation causes of points routinely. Pinpointing the supply of errors or discrepancies reduces the time to detection and system downtime. Much less want for reactive troubleshooting additionally interprets into improved useful resource utilization and operational value effectivity.
Evaluation of Guide Logs and Documentation
Through the years, loads of documentation round information integration workflows piles up throughout the group in inconsistent codecs and various places. AI-powered Pure Language Processing (NLP) methods can perceive, course of and interpret logs, documentation, and communication associated to information integration, and extract significant insights to detect points or establish areas for enchancment.
Information High quality Monitoring
Machine studying fashions could be educated to observe information for accuracy and completeness and routinely flag and handle information high quality points as they come up, typically with none human intervention
Automated Metadata Administration
AI-driven instruments can automate the gathering, tagging, and group of metadata associated to information integration processes. Information catalogs make it simpler to seek for and observe information lineage, dependencies, and different crucial data associated to information integration, selling higher information discovery and understanding.
Make Information Observability Integral to Your Trendy Information Integration Technique
Information observability, a notable innovation within the Gartner Hyper Cycle 2022, is quick attracting the eye of future-ready information engineers.
The ensuing explosion within the variety of observability options out there has led to a fragmentation of capabilities, with many merchandise defining information observability too narrowly, providing solely a subset of the required capabilities, or including to the complexity of the information integration ecosystem.
A complete observability resolution ought to provide end-to-end visibility, in addition to superior anomaly detection, predictive analytics, and automatic concern decision capabilities that work seamlessly throughout multi-cloud and hybrid cloud environments.
Nevertheless, this could not make life extra sophisticated for information engineers, who already need to handle and monitor various and sophisticated information pipelines.
To deal with this, fashionable information integration options are more and more embedding superior observability capabilities into the core product, which additional streamlines operations throughout the whole information provide chain.
AI-powered, end-to-end information administration and integration options will help you’re employed smarter at every stage of the information integration workflow whereas leveraging the advantages of superior information observability capabilities to scale back errors, handle prices and generate extra worth out of your information.
In regards to the creator: Sudipta Datta is a knowledge fanatic and a veteran product advertising supervisor. She has a wealthy expertise with information storage, information backup, messaging queue, information hub and information integration options in numerous firms like IBM and Informatica. She enjoys explaining advanced, technical and theoretical concepts in easy phrases and serving to prospects perceive what’s in it for them.
Associated Objects:
Information Observability ROI: 5 Key Areas to Assemble a Compelling Enterprise Case
There Are 4 Forms of Information Observability. Which One is Proper for You?
Observability Primed for a Breakout 2023: Prediction