Right here’s a painful reality: generative AI has taken off, however AI manufacturing processes haven’t stored up. In reality, they’re more and more being left behind. And that’s an enormous drawback for groups in every single place. There’s a need to infuse giant language fashions (LLMs) right into a broad vary of enterprise initiatives, however groups are blocked from bringing them to manufacturing safely. Supply leaders now face creating much more frankenstein stacks throughout generative and predictive AI—separate tech and tooling, extra information silos, extra fashions to trace, and extra operational and monitoring complications. It hurts productiveness and creates danger with a scarcity of observability and readability round mannequin efficiency, in addition to confidence and correctness.
It’s extremely onerous for already tapped out machine studying and information science groups to scale. They’re not solely being overloaded with LLM calls for, however face being hamstrung with LLM selections which will danger future complications and upkeep, all whereas juggling current predictive fashions and manufacturing processes. It’s a recipe for manufacturing insanity.
That is all precisely why we’re saying our expanded AI manufacturing product, with generative AI, to allow groups to securely and confidently use LLMs, unified with their manufacturing processes. Our promise is to allow your staff with the instruments to handle, deploy, and monitor all of your generative and predictive fashions, in a single manufacturing administration resolution that all the time stays aligned along with your evolving AI/ML stack. With the 2023 Summer time Launch, DataRobot unleashed an “all-in-one” generative AI and predictive AI platform and now you possibly can monitor and govern each enterprise-scale generative AI deployments side-by-side with predictive AI. Let’s dive into the small print!
AI Groups Should Deal with the LLM Confidence Drawback
Until you might have been hiding below a really giant rock or solely consuming 2000s actuality TV during the last yr, you’ve heard in regards to the rise and dominance of enormous language fashions. If you’re studying this weblog, chances are high excessive that you’re utilizing them in your on a regular basis life or your group has included them into your workflow. However LLMs sadly have the tendency to offer assured, plausible-sounding misinformation until they’re intently managed. It’s why deploying LLMs in a managed means is the very best technique for a corporation to get actual, tangible worth from them. Extra particularly, making them protected and managed with a view to keep away from authorized or reputational dangers is of paramount significance. That’s why LLMOps is vital for organizations in search of to confidently drive worth from their generative AI initiatives. However in each group, LLMs don’t exist in a vacuum, they’re only one sort of mannequin and a part of a a lot bigger AI and ML ecosystem.
It’s Time to Take Management of Monitoring All Your Fashions
Traditionally, organizations have struggled to watch and handle their rising variety of predictive ML fashions and guarantee they’re delivering the outcomes the enterprise wants. However now with the explosion of generative AI fashions, it’s set to compound the monitoring drawback. As predictive and now generative fashions proliferate throughout the enterprise, information science groups have by no means been much less geared up to effectively and successfully search out low-performing fashions which are delivering subpar enterprise outcomes and poor or detrimental ROI.
Merely put, monitoring predictive and generative fashions, at each nook of the group is vital, to scale back danger and to make sure they’re delivering efficiency—to not point out reduce guide effort that always comes with retaining tabs on rising mannequin sprawl.
Uniquely LLMs introduce a model new drawback: managing and mitigating hallucination danger. Basically, the problem is to handle the LLM confidence drawback, at scale. Organizations danger their productionized LLM being impolite, offering misinformation, perpetuating bias, or together with delicate info in its response. All of that makes monitoring fashions’ conduct and efficiency paramount.
That is the place DataRobot AI Manufacturing shines. Its intensive set of LLM monitoring, integration, and governance options permits customers to rapidly deploy their fashions with full observability and management. Whereas utilizing our full suite of mannequin administration instruments, using the mannequin registry for automated mannequin versioning together with our deployment pipelines, you possibly can cease worrying about your LLM (and even your traditional logistic regression mannequin) going off the rails.
We’ve expanded monitoring capabilities of DataRobot to offer insights into LLM conduct and assist determine any deviations from anticipated outcomes. It additionally permits companies to trace mannequin efficiency, adhere to SLAs, and adjust to pointers, guaranteeing moral and guided use for all fashions, no matter the place they’re deployed, or who constructed them.
In reality, we provide strong monitoring help for all mannequin sorts, from predictive to generative, together with all LLMs, enabling organizations to trace:
- Service Well being: Necessary to trace to make sure there aren’t any points along with your pipeline. Customers can monitor complete variety of requests, completions and prompts, response time, execution time, median and peak load, information and system errors, variety of shoppers and cache hit charge.
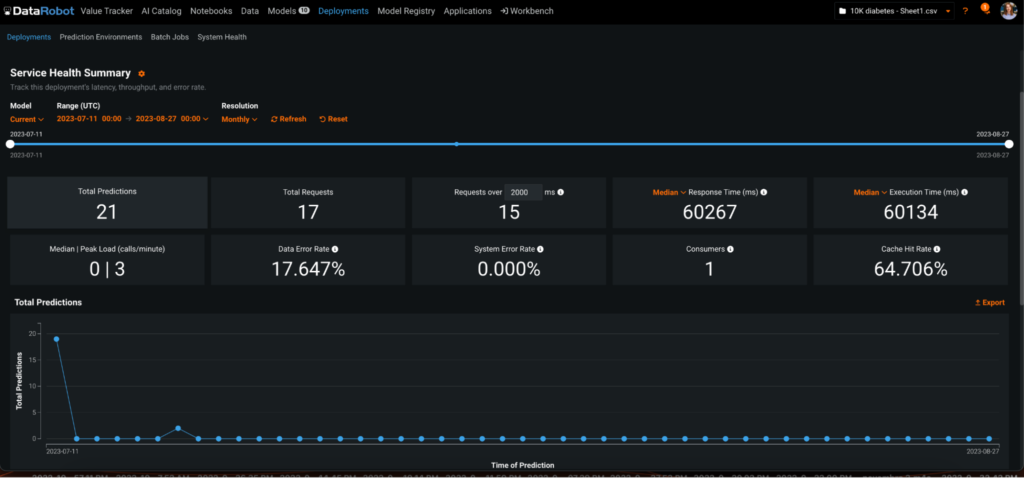
- Knowledge Drift Monitoring: Knowledge modifications over time and the mannequin you skilled a number of months in the past could already be dropping in efficiency, which will be pricey. Customers can monitor information drift and efficiency over time and might even monitor completion, temperature and different LLM particular parameters.
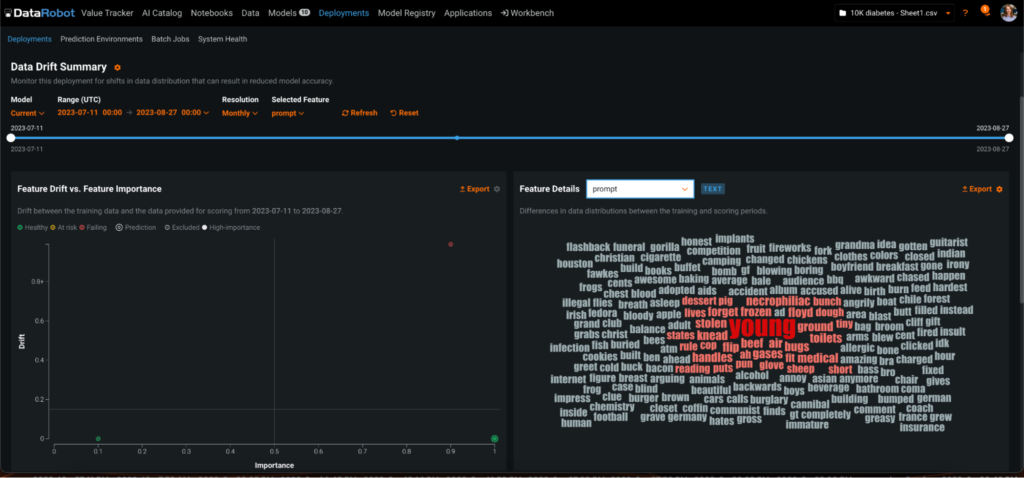
- Customized metrics: Utilizing {custom} metrics framework, customers can create their very own metrics, tailor-made particularly to their {custom} construct mannequin or LLM. Metrics reminiscent of toxicity monitoring, value of LLM utilization, and subject relevance can’t solely defend a enterprise’s repute but in addition be certain that LLMs is staying “on-topic”.
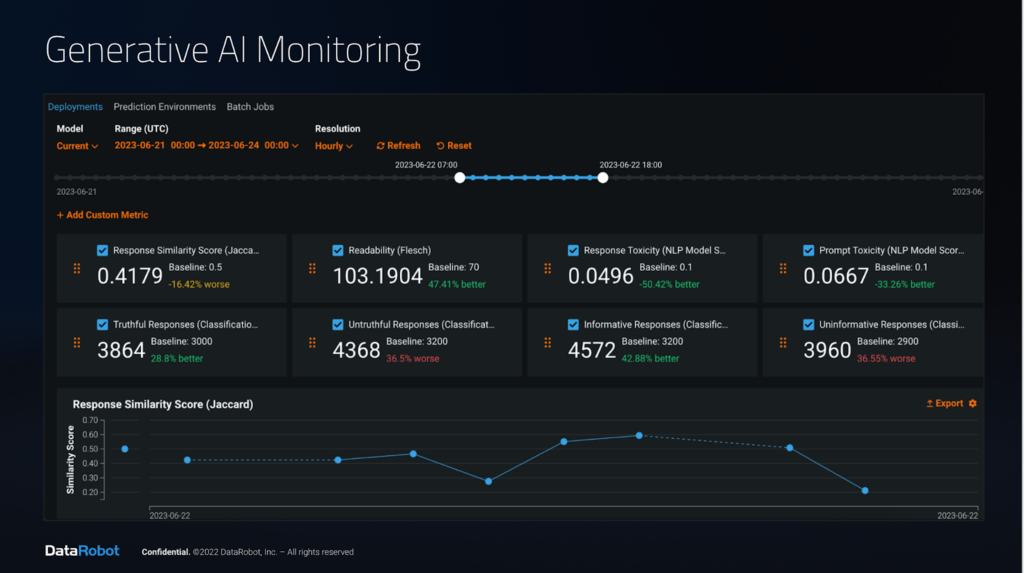
By capturing consumer interactions inside GenAI apps and channeling them again into the mannequin constructing part, the potential for improved immediate engineering and fine-tuning is huge. This iterative course of permits for the refinement of prompts based mostly on real-world consumer exercise, leading to more practical communication between customers and AI programs. Not solely does it empower AI to reply higher to consumer wants, however it additionally helps to make higher LLMs.
Command and Management Over All Your Generative and Manufacturing Fashions
With the frenzy to embrace LLMs, information science groups face one other danger. The LLM you select now is probably not the LLM you employ in six months time. In two years time, it might be an entire totally different mannequin, that you just need to run on a distinct cloud. Due to the sheer tempo of LLM innovation that’s underway, the chance of accruing technical debt turns into related within the area of months not years And with the frenzy for groups to deploy generative AI, it’s by no means been simpler for groups to spin up rogue fashions that expose the corporate to danger.
Organizations want a method to safely undertake LLMs, along with their current fashions, and handle them, monitor them, and plug and play them. That means, groups are insulated from change.
It’s why we’ve upgraded the Datarobot AI Manufacturing Mannequin Registry, that’s a elementary element of AI and ML manufacturing to offer a totally structured and managed strategy to prepare and monitor each generative and predictive AI, and your general evolution of LLM adoption. The Mannequin Registry permits customers to connect with any LLM, whether or not in style variations like GPT-3.5, GPT-4, LaMDA, LLaMa, Orca, and even custom-built fashions. It offers customers with a central repository for all their fashions, irrespective of the place they had been constructed or deployed, enabling environment friendly mannequin administration, versioning, and deployment.
Whereas all fashions evolve over time because of altering information and necessities, the versioning constructed into the Mannequin Registry helps customers to make sure traceability and management over these modifications. They’ll confidently improve to newer variations and, if essential, effortlessly revert to a earlier deployment. This stage of management is important in guaranteeing that any fashions, however particularly LLMs, carry out optimally in manufacturing environments.
With DataRobot Mannequin Registry, customers acquire full management over their traditional predictive fashions and LLMs: assembling, testing, registering, and deploying these fashions develop into hassle-free, all from a single pane of glass.
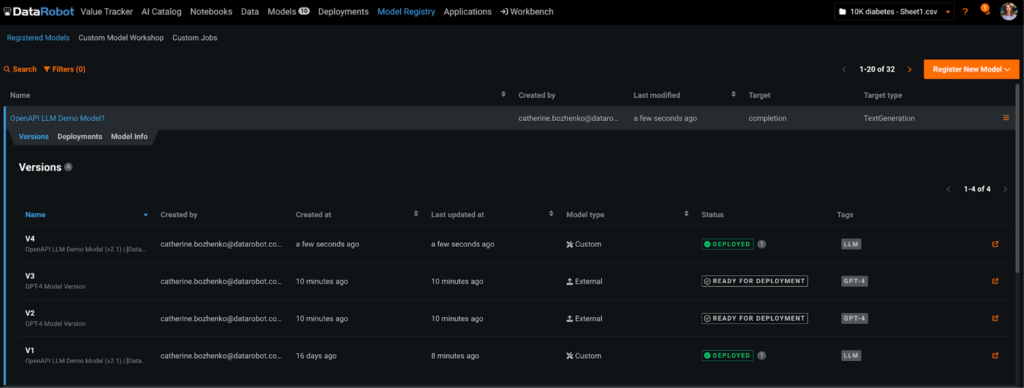
Unlocking a Versatility and Flexibility Benefit
Adapting to vary is essential, as a result of totally different LLMs are rising on a regular basis which are match for various functions, from languages to inventive duties.
You want versatility in your manufacturing processes to adapt to it and also you want the pliability to plug and play the correct generative or predictive mannequin on your use case moderately than making an attempt to force-fit one. So, in DataRobot AI Manufacturing, you possibly can deploy your fashions remotely or in DataRobot, so your customers get versatile choices for predictive and generative duties.
We’ve additionally taken it a step additional with DataRobot Prediction APIs that allow customers the pliability to combine their custom-built fashions or most popular LLMs into their purposes. For instance, it now makes it easy to rapidly add real-time textual content era or content material creation to your purposes.
You may also leverage our Prediction APIs to permit customers to run batch jobs with LLMs. For instance, if you want to robotically generate giant volumes of content material, like articles or product descriptions, you possibly can leverage DataRobot to deal with the batch processing with the LLM.
And since LLMs may even be deployed on edge gadgets which have restricted web connectivity, you possibly can leverage DataRobot to facilitate producing content material instantly on these gadgets too.
Datarobot AI Manufacturing is Designed to Allow You to Scale Generative and Predictive AI Confidently, Effectively, and Safely
DataRobot AI Manufacturing offers a brand new means for leaders to unify, handle, harmonize, monitor outcomes, and future-proof their generative and predictive AI initiatives to allow them to achieve success for at this time’s wants and meet tomorrow’s altering panorama. It allows groups to scalably ship extra fashions, irrespective of whether or not generative or predictive, monitoring all of them to make sure they’re delivering the very best enterprise outcomes, so you possibly can develop your fashions in a enterprise sustainable means. Groups can now centralize their manufacturing processes throughout their total vary of AI initiatives, and take management of all their fashions, to allow each stronger governance, and in addition to scale back cloud vendor or LLM mannequin lock-in.
Extra productiveness, extra flexibility, extra aggressive benefit, higher outcomes, and fewer danger, it’s about making each AI initiative, value-driven on the core.
To study extra, you possibly can register for a demo at this time from one in every of our utilized AI and product consultants, so you will get a transparent image of what AI Manufacturing can take a look at your group. There’s by no means been a greater time to begin the dialog and deal with that AI hairball head on.
Concerning the creator
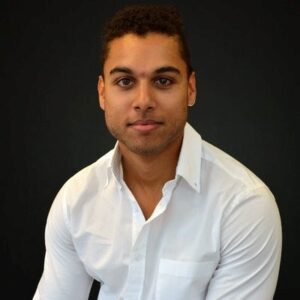
Brian Bell Jr. leads Product Administration for AI Manufacturing at DataRobot. He has a background in Engineering, the place he has led growth of DataRobot Knowledge Ingest and ML Engineering infrastructure. Beforehand he has had positions with the NASA Jet Propulsion Lab, as a researcher in Machine Studying with MIT’s Evolutionary Design and Optimization Group, and as an information analyst in fintech. He studied Laptop Science and Synthetic Intelligence at MIT.
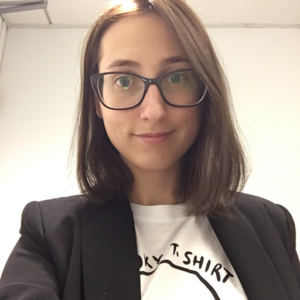
Kateryna Bozhenko is a Product Supervisor for AI Manufacturing at DataRobot, with a broad expertise in constructing AI options. With levels in Worldwide Enterprise and Healthcare Administration, she is passionated in serving to customers to make AI fashions work successfully to maximise ROI and expertise true magic of innovation.
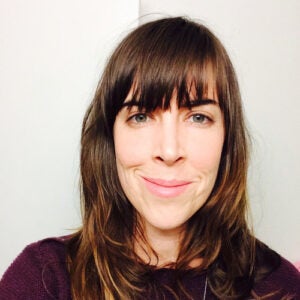
Mary Reagan is a Product Supervisor at DataRobot, and loves creating user-centric, data-driven merchandise. With a Ph.D. from Stanford College and a background as a Knowledge Scientist, she uniquely blends educational rigor with sensible experience. Her profession journey showcases a seamless transition from analytics to product technique, making her a multifaceted chief in tech innovation. She lives within the Bay Space and likes to spend weekends exploring the pure world.