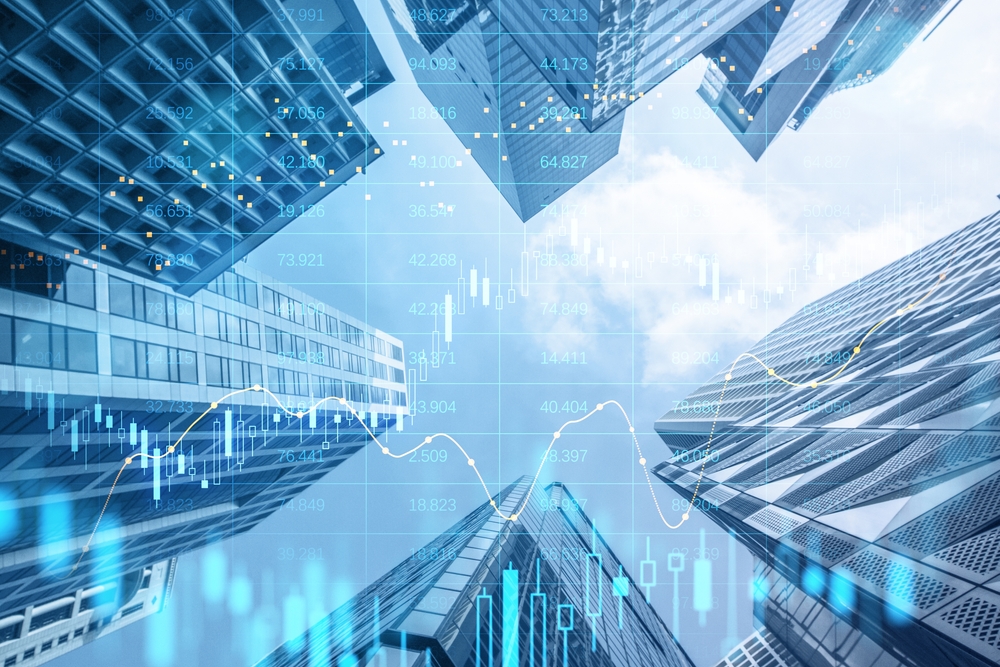
(Golden Dayz/Shutterstock)
Knowledge and enormous language fashions (LLMs) can save banks and different monetary companies hundreds of thousands by enhancing automation, effectivity, accuracy, and extra. McKinsey experiences that the productiveness elevate from generative AI can result in a rise of 3-5% of annual income within the banking sector, which is equal to $200 billion to $340 billion of further annual income.
Given the big quantity and number of knowledge that’s accessible within the monetary business, LLMs can deliver important value-add to monetary companies companies. Under are just some examples of how Gen AI and LLMs can assist monetary companies.
Fraud Prevention: Generative AI is on the forefront of growing cutting-edge fraud detection mechanisms. By analyzing huge knowledge swimming pools, it might discern intricate patterns and irregularities, providing a extra proactive strategy. Conventional methods, typically overwhelmed by the sheer quantity of information, would possibly produce false positives. Generative AI, in distinction, constantly refines its understanding, lowering errors and making certain safer monetary transactions.
Compliance:Â Anti-Cash Laundering (AML) laws are vital in sustaining the integrity of monetary methods. Generative AI simplifies compliance by sifting by intricate transactional knowledge to pinpoint suspicious actions. This not solely ensures monetary establishments adhere to world requirements but additionally considerably reduces the probabilities of false positives, streamlining operations. A Generative AI mannequin will help create extra environment friendly screening and quicker doc evaluation, together with monitoring and reporting duties.
Knowledge-driven decision-making: Given the potential to work with unstructured textual content knowledge, LLMs are in a position to attract insights from knowledge sources reminiscent of information experiences, social media content material, and publications. This permits firms within the monetary business to attract from underutilized sources.
Buyer interplay and assist: LLMs have boosted the capabilities and expectations we have now round chatbots and digital assistants. LLM-powered chatbots reminiscent of ChatGPT have proven an immense capability for human-like communication experiences. Incorporating these chatbots into monetary buyer assist companies will enhance the effectivity and the character of buyer interactions. For example, a digital private adviser who can present tailor-made perception into investments or private monetary administration may be extraordinarily well-received by clients.
Enterprise innovation and effectivity: We’ve got lately seen a surge of LLM-based add-ons for present instruments and applied sciences. For example, pure language-based directions, programming assistants, and writing assistants have gotten extraordinarily frequent. These LLM-based functionalities can result in important innovation and effectivity to the finance business.
What Are the Challenges?
Coaching LLMs with monetary knowledge: LLMs are presently skilled on the Web. Monetary companies use circumstances would require fine-tuning these fashions with use case-specific monetary knowledge. New entrants will most likely begin refining their fashions with public firm financials, regulatory papers, and different sources of simply accessible public monetary knowledge earlier than ultimately utilizing their very own knowledge as they accumulate it over time.
Present gamers, like banks or giant platforms with monetary companies operations, can leverage their present and proprietary knowledge, probably giving them an preliminary benefit. Present monetary companies firms, nevertheless, are usually overly conservative in the case of embracing giant platform shifts. This doubtless offers the aggressive edge to unencumbered new entrants.
Mannequin output accuracy: Given the affect the reply to a monetary query can have on people, firms, and society, these new AI fashions must be as correct as doable. They will’t hallucinate or make up mistaken however confident-sounding solutions to vital questions on one’s taxes or monetary well being, they usually must be way more correct than the approximate solutions for fashionable tradition queries or generic highschool essays. To start out, there’ll typically be a human within the loop as a ultimate verification for an AI-generated reply.
Bias Amplification: AI fashions, as refined as they’re, nonetheless depend on human-generated coaching knowledge. This knowledge, with its inherent biases—whether or not intentional or not—can result in skewed outcomes. For example, if a selected demographic is underrepresented within the coaching set, the AI’s subsequent outputs might perpetuate this oversight. In a sector like finance, the place fairness and equity are paramount, such biases might result in grave penalties. Monetary leaders must be proactive in figuring out these biases and making certain their datasets are as complete and consultant as doable.
Knowledge Privateness & Compliance: Defending delicate buyer knowledge stays a major concern with generative AI purposes. Guaranteeing the system adheres to world requirements just like the Normal Knowledge Safety Regulation (GDPR) and the California Shopper Privateness Act (CCPA) is essential. AI could not inherently know or respect these boundaries, so its use should be moderated with stringent knowledge safety tips, significantly within the monetary sector the place confidentiality is paramount.
High quality of Enter Knowledge: Generative AI is barely nearly as good as the info fed to it. Inaccurate or incomplete knowledge can inadvertently result in subpar monetary recommendation or choices.
LLMs will improve effectivity by automating and streamlining quite a lot of duties. It’ll allow firms to make higher sense of information, significantly unstructured textual content knowledge, thereby permitting for extra knowledgeable decision-making. With greater pure language processing capabilities led by LLMs, customer-oriented instruments reminiscent of chatbots shall be extra able to taking up a bigger portion of buyer assist, in addition to offering improved assist companies. This can enhance the standard of buyer expertise whereas releasing up priceless human time and capability to have interaction in additional value-generating duties.
Concerning the Creator: Namrata Ganatra, Chief Product and Know-how Officer of Pipe has over a decade of expertise and a confirmed observe document of main profitable initiatives and contributing to the expansion of a number of notable firms in fintech, AI, and crypto. Namrata’s latest expertise contains main product and engineering at Autograph, a number one NFT platform. She additionally based a Generative AI startup that helped SMBs develop multi-channel e-commerce gross sales, which was efficiently acquired by Thrasio. Previous to that, Namrata held senior roles at Coinbase and Fb, the place she performed a vital position in shaping cost technique and scaling cost infrastructure to satisfy the wants of hundreds of thousands of customers. Namrata can also be an Angel Investor and Advisor at Distyl AI, which has partnered with OpenAI to construct Generative AI Options for Enterprises.
Associated Objects:
How Giant Language Fashions and People Can Make Strategic Choices Collectively
GenAI Hype Bubble Refuses to Pop
LLMs Are the Dinosaur-Killing Meteor for Outdated BI, ThoughtSpot CEO Says
Â